
Now we’ve got that out of the way, it’s time to move on to the main course of this article.
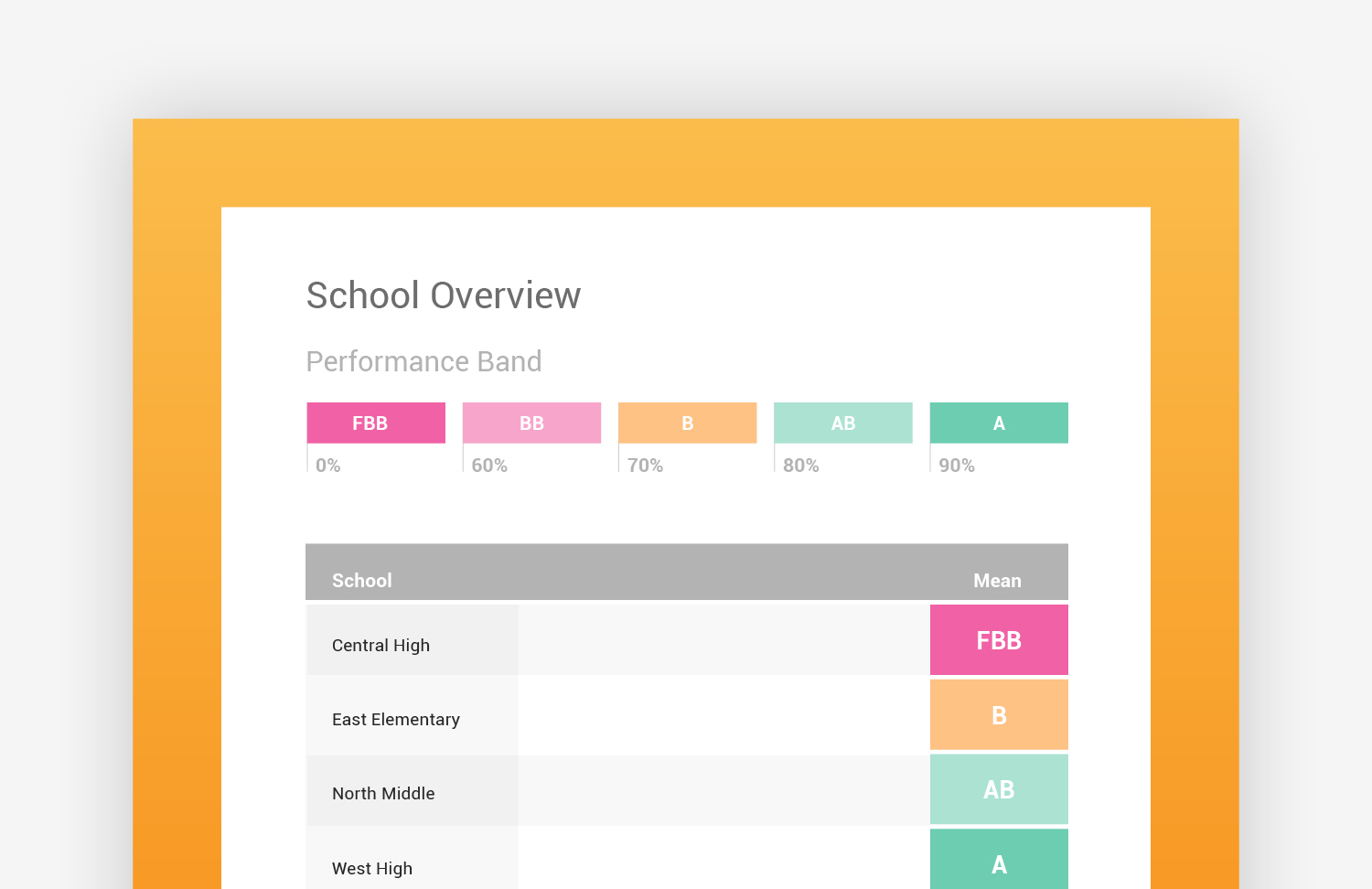
A high response rate (>80%) from a small, random sample is preferable to a low response rate from a large sample. Nevertheless, in spite of these recent research studies, a higher response rate is preferable because the missing data is not random. However, recent studies have shown that surveys with lower response rates (near 20%) had more accurate measurements compared to surveys with higher response rates (near 60 or 70%). Therefore, non-response bias may make the measured value for the workload too low, too high, or, if they balance each other out, “right for the wrong reasons.”Ī survey’s response rate has always been viewed as an important indicator of survey quality. If you select a sample of 1000 managers in a field and ask them about their workload, the managers with a high workload may not answer the survey because they do not have enough time to answer it, and/or those with a low workload may decline to respond out of fear that their supervisors or colleagues will perceive them as surplus employees. A low response rate can give rise to sampling bias if the nonresponse is unequal among the participants regarding the outcome. Ryan, David Madigan, and George Hripcsak. Suchard, Jianxiao Yang, Yuxi Tian, Alejandro Schuler, Patrick B. The OHDSI community believes in the values of open science and transparency, and all results are publicly available in its GitHub repositories.Īdditional authors are Soledad Cepeda, Marc A. The methods evaluated in this paper are part of the OHDSI Methods Library ( ), a set of open-source R packages that is available for all data standardized in the OMOP Common Data Model.
BENCHMARK RESEARCH STUDIES SERIES
Particularly self-controlled designs such as the self-controlled case series performed best in many scenarios, although there is no silver bullet. Through the Benchmark, the researchers were able to show that using empirical calibration it is possible to distinguish between study designs that merely produce noise and those that are informative. “For every study, we need to measure the potential for bias through the use of controls, and calibrate our estimates accordingly.” “Our results show that simply assuming that an observational study design will produce the right answer is little more than wishful thinking,” Schuemie says.
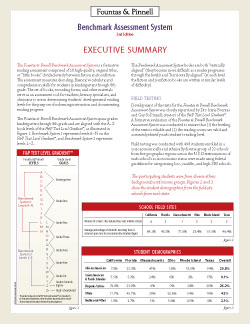
How can we trust in observational findings moving forward? One solution is a technique developed within OHDSI called ‘empirical calibration’ ( ), which adjusts the results and the confidence we can have in the results based on what was observed for a set of control questions. Selection bias, confounding, and misspecification are among the sources of systematic error that plagues the validity of potentially important findings within the healthcare community.
BENCHMARK RESEARCH STUDIES SOFTWARE
Using both negative and positive controls (questions where the answer is known), a set of metrics and open-source software tools developed within the OHDSI community, the research team determined that most commonly used approaches to effect-estimation observational studies are falling short of expected confidence levels.
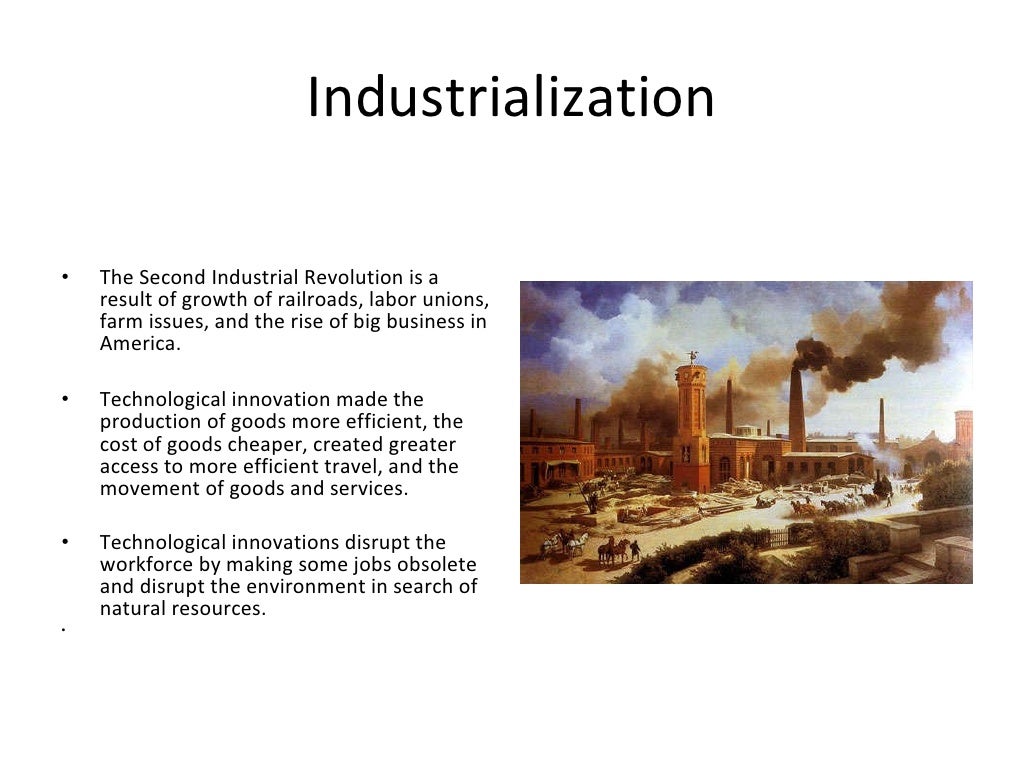
This paper presents the OHDSI Methods Benchmark to evaluate five methods commonly used in observational research (new-user cohort, self-controlled cohort, case-control, case-crossover, and self-controlled case series designs) over a network of four large databases standardized to the OMOP Common Data Model. Led by Martijn Schuemie, OHDSI researchers recently published “How Confident Are We About Observational Findings in Healthcare: A Benchmark Study” in the Harvard Data Science Review to tackle this important issue. We need to answer the question “to what extent can we trust observational research?” However, confidence in the results of such observational research is typically low, for example, because different studies on the same question often produce conflicting results, even when using the same data. The prevalence of electronic healthcare data allows researchers the opportunity to study the effects of medical treatments.
